Good Today, Better Tomorrow
Elevate was founded on a legacy of data and innovation coupled with a customer-first approach. That’s why we’re committed to providing solutions for non-prime customers today, and have never lost sight of our purpose of helping them on their way to a better financial future. We call our approach “Good Today, Better Tomorrow.”
Our Solutions
Our innovative, online tech-enabled credit solutions are built based on our simple mission: “Good Today, Better Tomorrow.” They provide immediate relief to customers today and can help them build a brighter financial future. By utilizing technology and advanced analytics, we are able to provide customers with access to competitively priced credit when they need it. And we offer a number of financial wellness and consumer-friendly features that are unmatched in the non-prime lending market.
Our
Corporate
Values
Elevate is guided by a set of corporate values to ensure we provide quality lending solutions that fit the needs of our customers. Every Elevate Employee is held accountable to these 4 core values:
Think Big
As industry innovators, creative thinking is our competitive advantage. Together we share a responsibility to think outside the box and embrace change.
Do the right thing
Do the right thing is not optional. We hold each other to the highest standards and earn our reputation every day.
Raise the Bar
Excellence isn't a skill, it's a habit, born for a continuous drive to do better. We embrace this as a company and as individuals, by building on successes and learning from failures.
Win together
Our goals are too big to achieve as individuals. Collaboration isn't a byproduct of our work, it is our primary focus. It's also more fun.
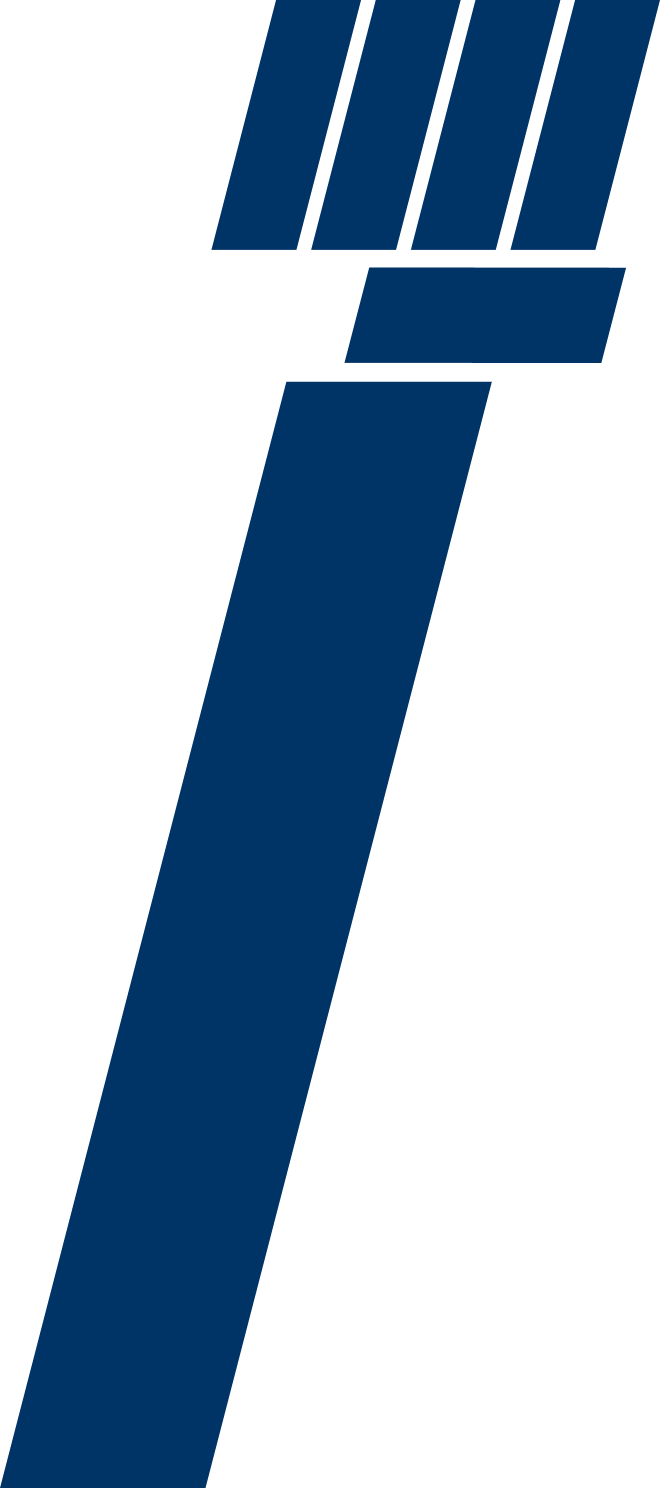
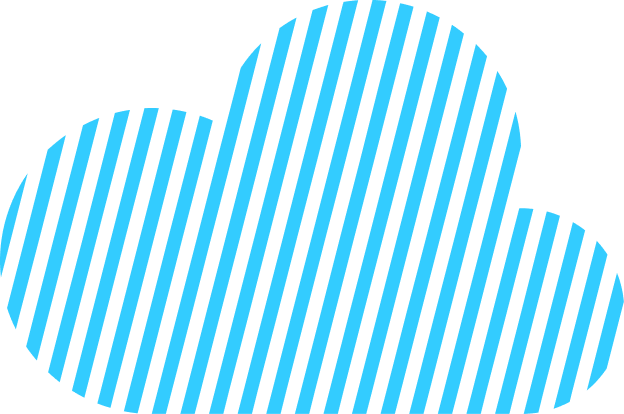
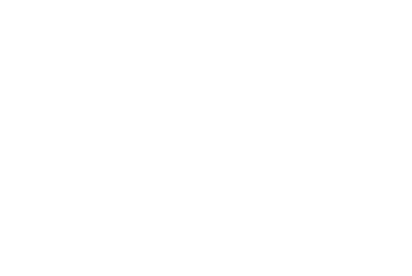
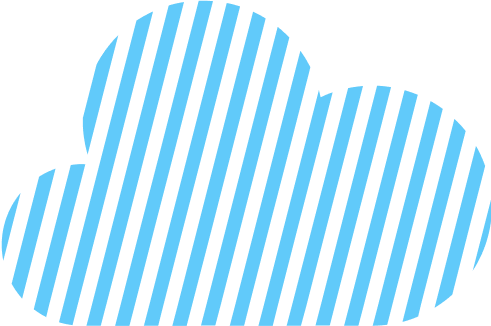